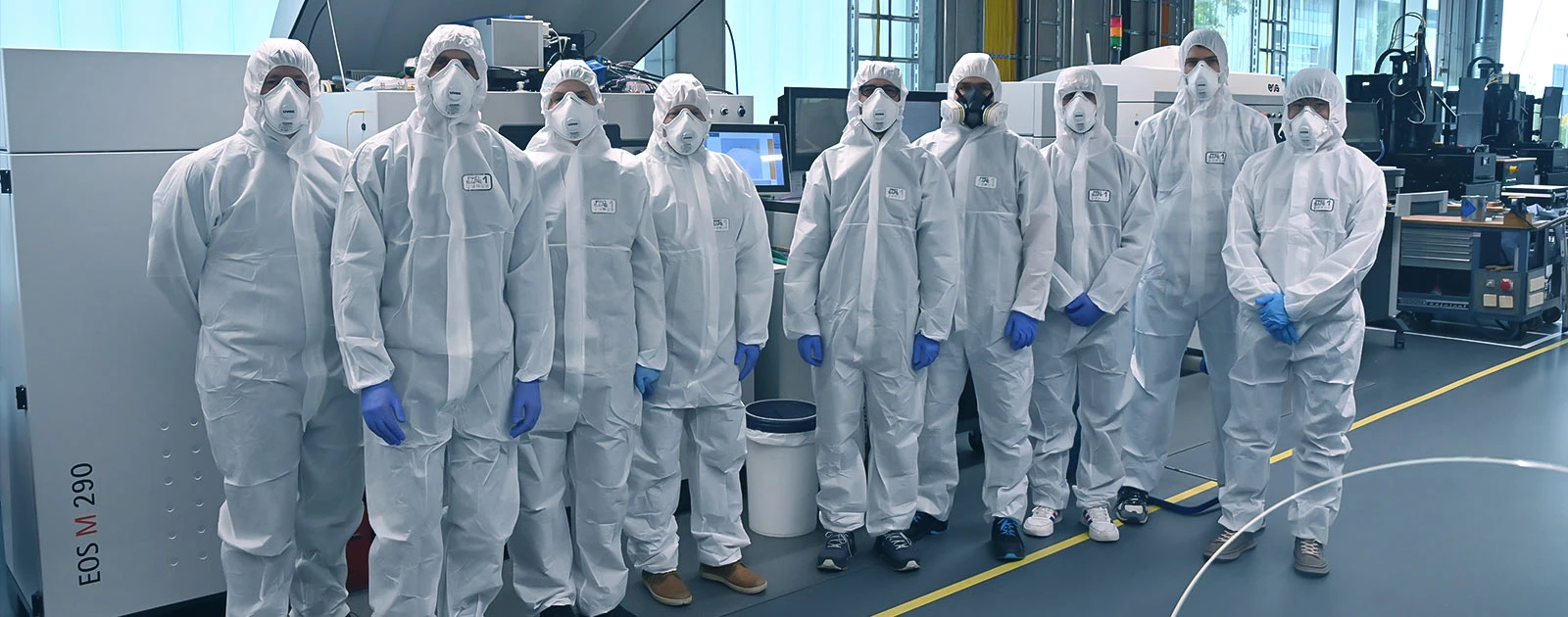
Machine learning for more quality in additive manufacturing
Additive manufacturing offers unprecedented flexibility in the production of complex geometries, but is susceptible to defects during the production process. With the ML-S-LeAF project, we have made a decisive contribution to the development of new possibilities for digital quality assurance.
ML-S-LeAF stands for an innovative approach to quality assurance in additive manufacturing. The project, which is funded by the German Federal Ministry for Economic Affairs and Climate Protection, aims to make manufacturing processes more resource-efficient.
The aim of the research consortium is to develop an automated process monitoring system based on simulated noise emissions during an industrial printing and melting process.
Project partners
Together with a top-class consortium from industry and science, we worked on pioneering quality assurance methods:
Fraunhofer IDMT
Experts for digital media technologiesSAM and PTW at TU Darmstadt
Cutting-edge research in acoustics and production technologyOmegaLambdaTec GmbH
Specialists for data analysis and machine learningNovicos GmbH
Acoustics experts for simulation and measurement
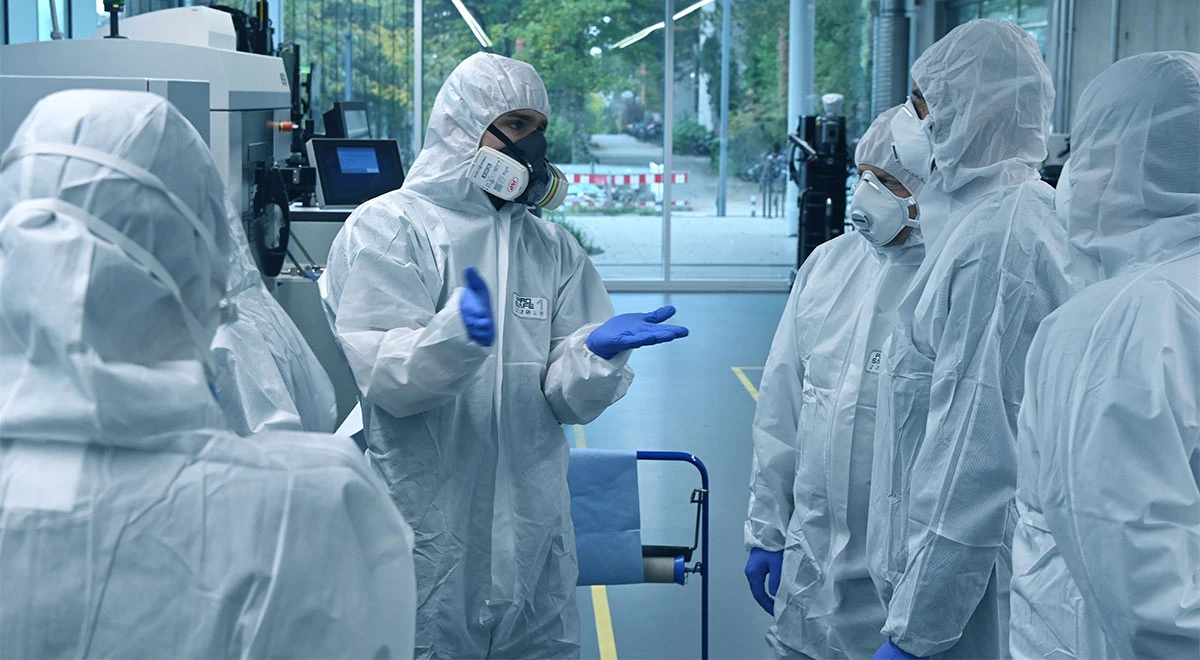
Thanks to all sponsors
We would like to thank our consortium partners as well as the BMWK (still BMWi at the time of application) and Forschungszentrum Jülich for the funding and support of this successful project.
The project created the basis for a digital, AI-based quality assurance system that was developed specifically for use in lightweight construction. This represents a significant step towards the complete digitalization of quality monitoring in additive manufacturing.
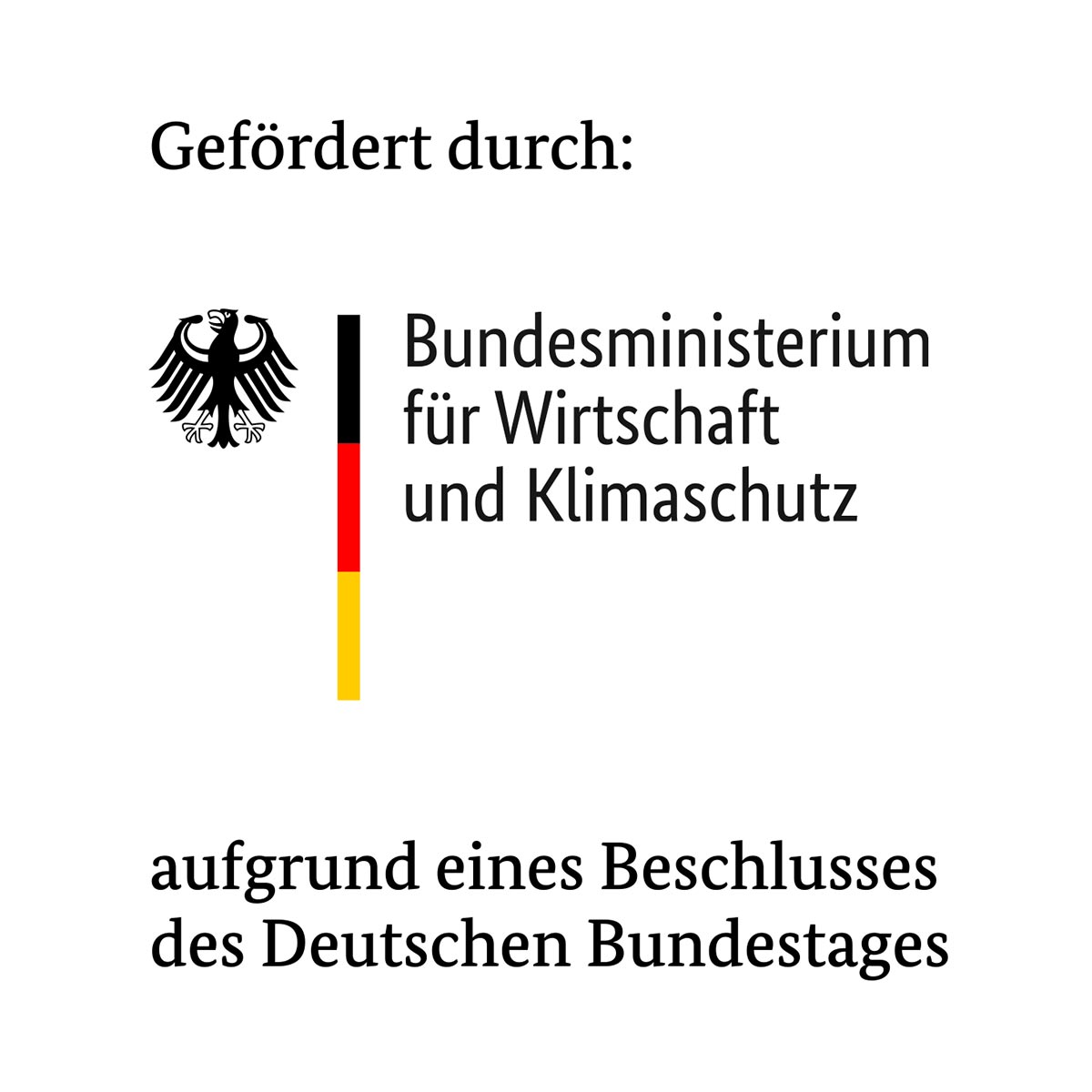
Your contact
I will be happy to answer any questions you may have and provide further details on the implementation and results of ML-S-LeaF.
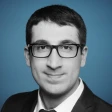
Dr.-Ing. Ömer Yildiz
Further details
We continuously share the ongoing developments and successes of research projects such as ML-S-LeAF on LinkedIn. Follow us to get the latest updates and learn how machine learning is revolutionizing the manufacturing industry.
Novicos on LinkedIn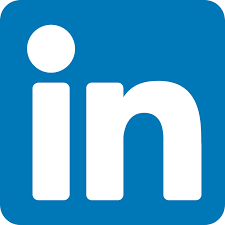